Start your DSaaS Journey Today!
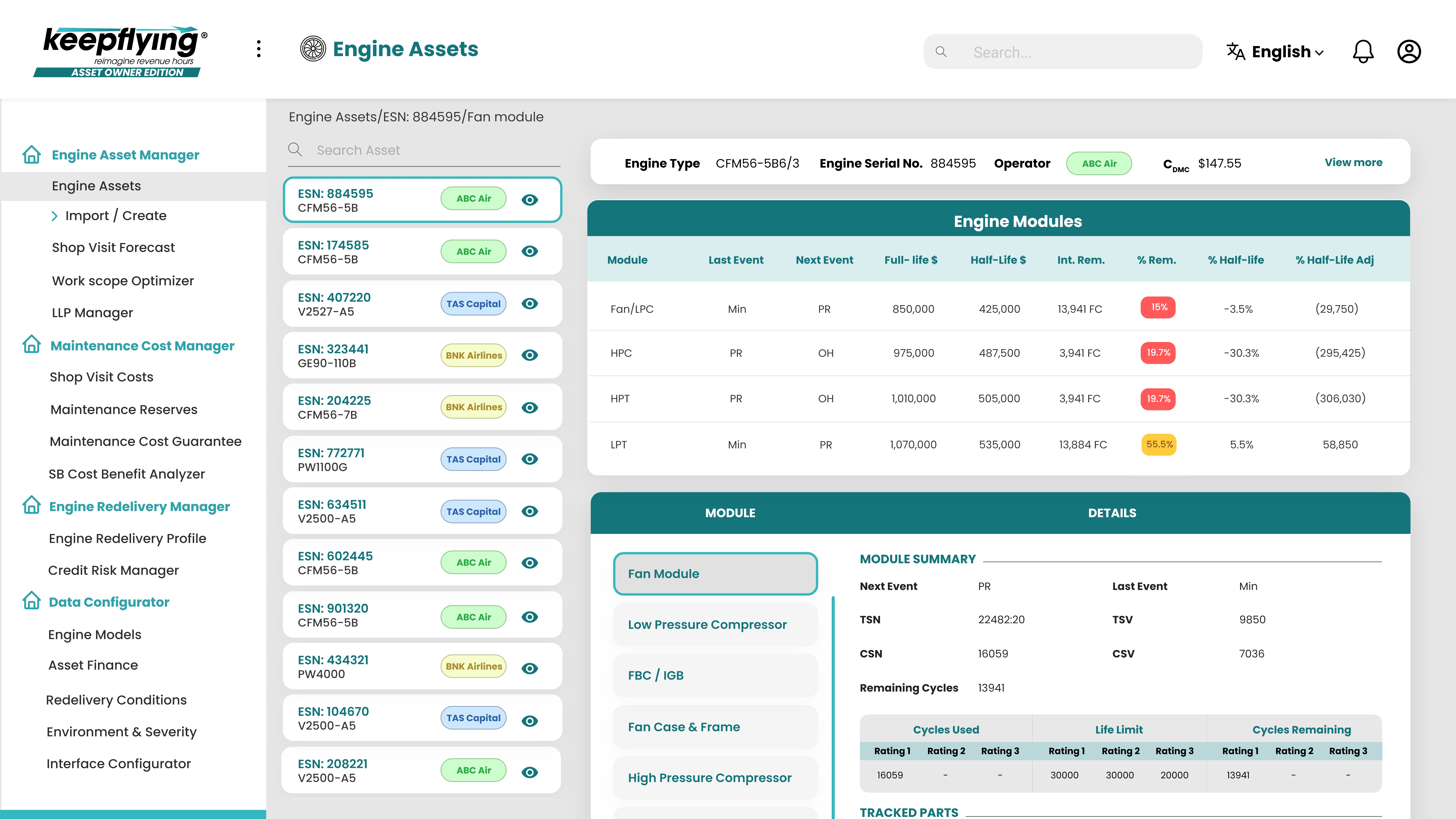
Using Generative AI and Large Language Models, we have significantly streamlined data wrangling and analytics in aviation, traditionally characterized by disorganized and uncatalogued information. Our innovative approach has successfully transformed unstructured data, including descriptive texts and scanned images, into valuable, structured data, marking key milestones in this field.
Expected to contribute $2.6 to $4.4 trillion annually to the global economy, impacting sectors like high tech, retail, banking, and more
By Mckinsey & Company
Transforming Aviation Data Management with Large Language Models (LLMs)
LLMs employ artificial intelligence to deeply analyze vast text datasets, efficiently extracting critical information such as Engine Serial Numbers, Total Time, and Cycles from complex aviation maintenance reports.
Even when faced with scattered or poorly formatted information, LLMs adeptly restructure and clarify these data sets, turning what was once noisy and unstructured into valuable, organized key-value pairs.
This capability is not just an improvement but a revolution in data extraction and management, significantly enhancing accuracy and efficiency in the aviation sector.
In the fast-paced world of aviation, data updates occur almost in real-time. Efficiently managing this data is crucial for accurate analytics. This is where Large Language Models (LLMs) play a transformative role.
Aviation data is dynamic, requiring continuous updates. Traditional web scraping methods struggle to keep pace, but LLMs provide a more efficient solution for capturing these rapid changes.
Traditional data sourcing methods are often rigid, demanding specific logic tailored to each website's unique design. LLMs, however, introduce flexibility, allowing for a more generalized approach that adapts to varying website architectures and designs.
The use of LLMs in data extraction ensures resilience against frequent changes in website structures. This results in more stable and reliable data sourcing for aviation analytics, ensuring continuity and accuracy in a field where information is constantly evolving.
Low Rank Adaptation (LoRA) provides an efficient algorithm to fine tune large language models for specific purposes. LoRA algorithm is based on matrix decomposition; it reduces the matrix of billion parameters into smaller ones which reduces the size and computations required by the model.
Large Language Models (LLMs) can exhibit unpredictable behavior, posing risks to business performance and trust. At KeepFlying®, we mitigate these challenges with advanced monitoring tools, ensuring reliable, secure, and compliant use of LLMs in our cutting-edge applications.
Our leading-edge LLM Document Classification system, powered by OpenAI's GPT-3.5, employs Few-shot Learning and Prompt Engineering for rapid, accurate categorization of aviation documents. This precise system effectively sorts Technical Logs, Statements, and more, enhancing classification accuracy through finely-tuned LLM interactions.
Our innovative hybrid system merges Machine Learning and Large Language Models to efficiently extract Non-Incident Statements from Engine Minipacks, enhancing aviation safety. It features a two-layer approach: the first layer uses ML for initial extraction, while the second layer employs LLMs for advanced language comprehension and clear summarization of data.
Our specialized Aviation Question Answering System for Engine Minipacks rapidly delivers precise information from engine documents. Utilizing a Retrieval-Augmented Generation (RAG) framework with Large Language Models and a vector database, it intelligently interprets queries and sources contextually relevant documents, ensuring detailed and accurate responses.
We've introduced Aviation LLM Agents, a revolutionary approach to managing Engine Minipacks. These agents do more than generate text; they interactively respond to user queries, perform tasks, and access a wealth of information through OCR and a Vector Database, significantly enhancing the way aviation professionals handle critical data.